A Brief Journey Through Data Collection, Labelling, and Annotation for Computer Vision
In the fast-evolving landscape of artificial intelligence and machine learning, the crucial role of high-quality training data cannot be overstated. As this year we celebrate the 2-year anniversary of “Training Data”, a pioneering company in the realm of data collection, labelling, and annotation for computer vision, it’s a perfect opportunity to reflect on the remarkable journey this company has undertaken and the significant impact it has had on the AI industry.
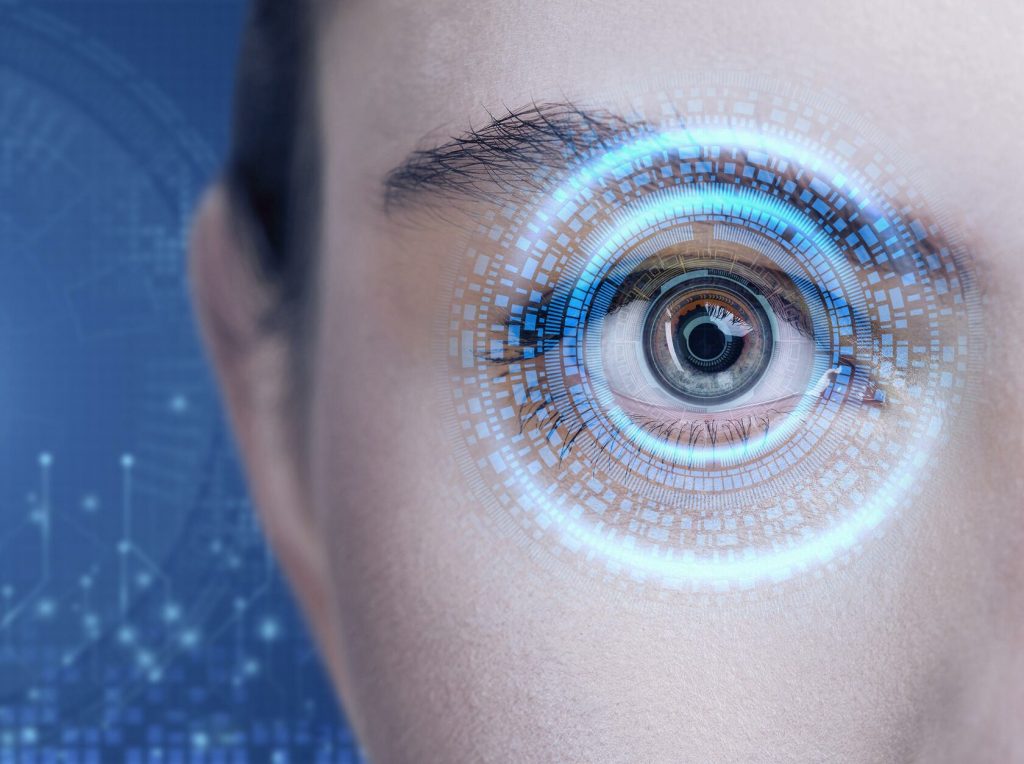
How it all began?
The development of data collection and annotation services can be traced back to the emergence of machine learning and artificial intelligence as fields of study and practical application. The concept of data collection and annotation has evolved over time as the demand for accurately labeled data to train AI models has grown.
1950s-1960s: Early Machine Learning
During this period, researchers were laying the groundwork for machine learning and AI. While the concept of data collection and annotation services wasn’t fully formed, efforts were being made to collect and organize data for early computational models.
1970s-1980s: Growth of Databases
The emergence of structured databases and relational database management systems in this era facilitated more organized data storage and retrieval. Data was being collected and stored digitally, paving the way for more systematic approaches to data handling.
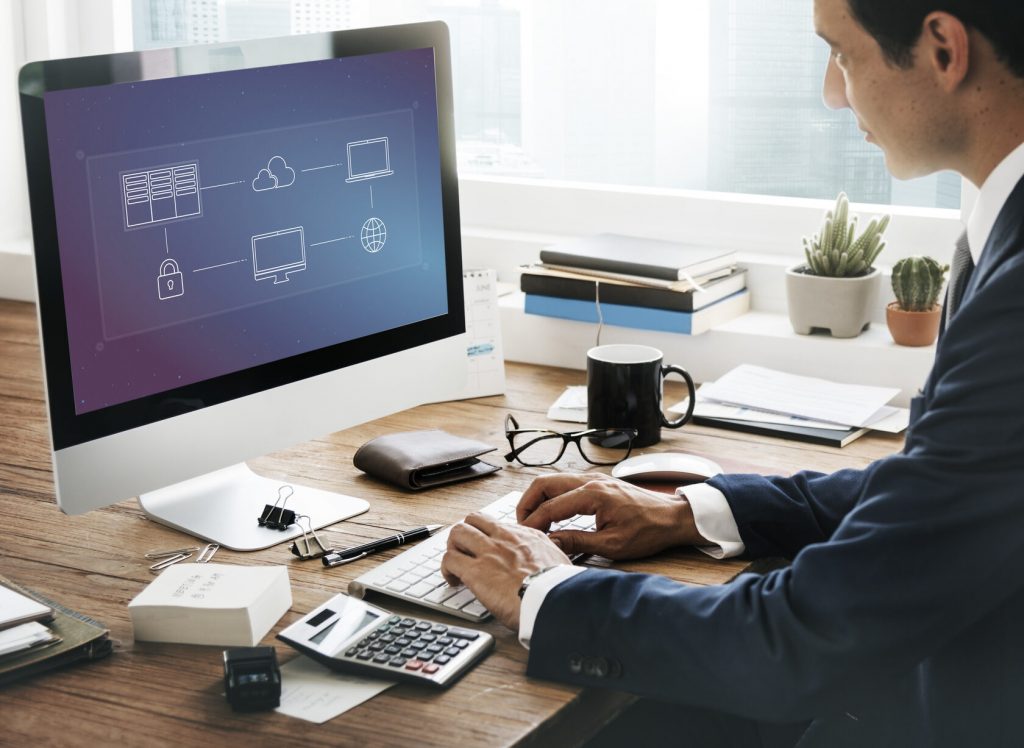
1990s: Expansion of the Internet
The widespread adoption of the internet led to an increase in digital data availability. Researchers and businesses started collecting data from online sources, setting the stage for the need for curated and annotated data.
2000s: Crowdsourcing and Web 2.0
The advent of Web 2.0 brought about user-generated content and the rise of crowdsourcing platforms. Amazon Mechanical Turk, launched in 2005, played a role in the early crowdsourced data annotation efforts. This laid the foundation for more systematic approaches to data labeling.
2010s: Proliferation of Machine Learning
The explosion of machine learning and AI applications in the 2010s marked a turning point. As models became more complex and required larger and more diverse datasets, the need for high-quality annotated data became apparent. Companies started offering specialized data collection and annotation services to meet this demand.
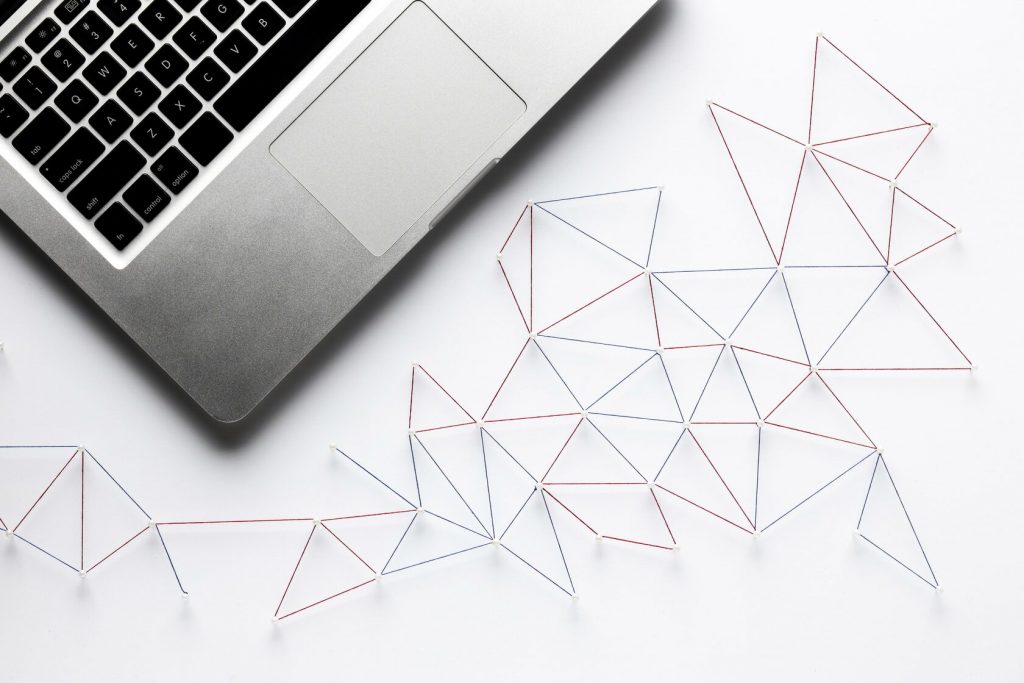
2010s-Present: Specialized Data Annotation Companies
Starting in the mid-2010s, companies began to emerge with a specific focus on providing data annotation and labeling services. These companies recognized the challenges associated with creating accurate and diverse training datasets for AI models. They combined crowdsourcing, automation, and human expertise to deliver high-quality labeled data for a wide range of applications.
Throughout this timeline, the development of data collection and annotation services was closely tied to the evolution of AI technologies, the increasing complexity of AI models, and the recognition of the critical role that labeled data plays in the success of machine learning projects. As the AI landscape continues to evolve, data collection and annotation services remain essential to training robust and accurate AI models across various domains
Present Time: Major Trends in Data Annotation
Interesting innovations and approaches are emerging in the field of data annotation. Among others, synthetic datasets, pre-labeling, and the human in the loop approach are actively discussed today.
Synthetic Datasets
When collecting real data is challenging or expensive, synthetic datasets are often used. This allows to generate datasets of any size.
Pre-labeling
In this case, data is processed using a predictive neural network, and annotators only need to make minor adjustments to these results. This speeds up labeling by up to 10 times.
Human In The Loop
This concept combines artificial and human intelligence. It is typically implemented in companies where the cost of errors is very high to achieve the highest level of accuracy. In this scenario, machine learning (ML) performs most of the tasks, while annotators label the most complex cases. All the data annotated by annotators is also added to the training dataset, and the model undergoes retraining every week.