I Want to Work in IT: Why this Message doesn’t Work in the Field of Data Annotation
Anastasia Stoyanova, HR and L&D Manager at Training Data
The data annotation market grew by 70% in 2022, indicating the rapid development of the AI industry and making it a promising direction for labor market growth. With the emergence of companies specializing in data annotation, new professions (such as data quality control manager, data annotation project manager, data annotator) have emerged, which candidates were not familiar with before.
In mid-July 2022, the HR department received a request to hire several project managers due to an increase in data annotation projects. At that time, the team already had more than 15 project managers, whose regular tasks included:
- Communication with clients at all stages of project implementation regarding project conditions, deadlines, data extraction, adjustments to the technical task, etc.
- Compilation and execution of pilot projects to determine metrics and the cost of the order.
- Preparation of detailed technical tasks for data annotators, their training and certification.
- Quality control and project deadline monitoring.
- Data transfer, summarizing and completing the work cycle with the client.
- Keeping various project reports and calculating the salary of their team.
Due to the novelty of the position, there were no requirements for candidates to have experience in the position of a data annotation project manager. The focus was on finding project managers with skills in organizing processes, managing a team, and the ability to independently master new tools and areas of knowledge.
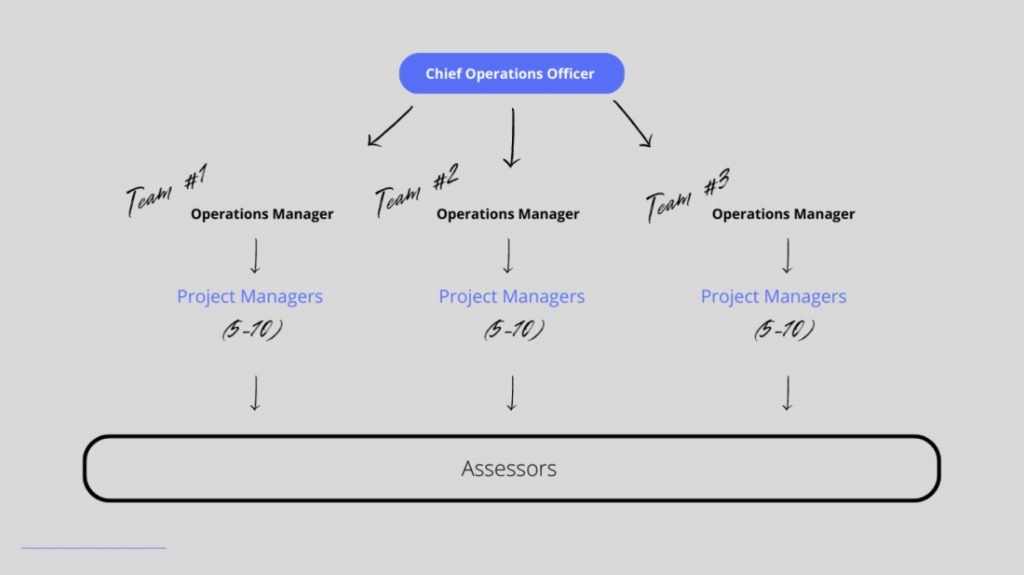
Evaluation criteria for applicants
HR, together with operational managers, developed a strategy for evaluating candidates for project manager positions. The assessment of applicants was planned in 4 stages:
1. Resume screening.
2. Motivational questionnaire.
3. A short questionnaire where applicants were asked to talk about:
- Their experience as project managers.
- Specialization in education.
- Remote work experience.
- Effective presentation skills
- Salary expectations
4.Management cases.
Alongside the questionnaire, applicants were asked to solve three cases aimed at verifying skills in organizing processes, communicating with clients, finding solutions in complex stressful situations, and general resourcefulness. Here are a few examples of the test task:
“You have been given a project with a large volume of data to process – 500,000 photos. The deadline is in one week. The average speed of one performer is 800 photos per hour. Performers rarely work more than 4 hours a day. The project needs to start tomorrow. How will you organize the working process? What will be your steps in preparing the team, managing the project, monitoring, and delivering it?
During the first and second stages, applicants received a test task on CVAT—one of the main data annotation programs. They were required to familiarize themselves with the program’s algorithms, and in the open version, create a task with 5 photos of cats, creating a polygon on each with two eyes and a nose. Afterward, they were to send the backup to HR.
5.Interview with HR and operational managers.
According to HR forecasts, there was expected to be a moderate number of responses to this vacancy since data annotation is a narrow specialization, and not all applicants may be suitable for the fast-paced work environment in the company. While the first three cases can be logically solved (based on experience), the fourth case may not be clear even at the reading stage (although it is basic for our vacancy).
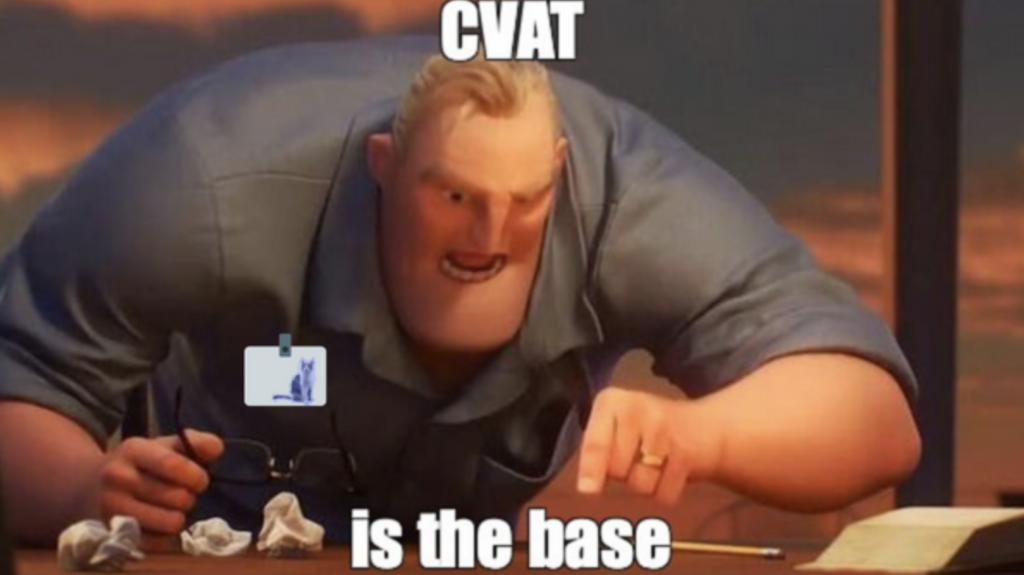
The outcomes
In reality, the number of responses was significantly higher than HR’s expectations. Within the first day after posting the vacancy, there were 308 responses, and within two days, it exceeded 600. To be honest, the batch of responses for the next two days and beyond was not thoro ughly processed, as the vacancy was closed successfully within the first three hundred.
Due to the large number of responses on the first day, it was necessary to slightly adjust the applicant evaluation strategy, specifically by removing the initial resume screening. Firstly, this significantly increased the response processing time, and secondly, there was a fear of losing good candidates due to a focus on specific experience. Resume screening was done after analyzing the questionnaire and cases.
295 applicants filled out the questionnaire, resulting in a 95.8% conversion rate. It was found that about 20 people, when asked about their “areas of immediate development,” saw themselves as developers, programmers, or leaders of IT teams. Below are examples of applicants’ responses:
“I already work in the IT sphere, I am studying to become a Frontend developer.”
“This is definitely development in the IT industry, related to product, project, analytics, and programming.”
“IT industry.”
“I am mainly interested in the IT sphere and its part dedicated to development management. I would like to develop as a project manager in an IT team.”
“My goal is to get an offer for a PM position, advance in studying the IT direction (I’m learning frontend, currently at the basic level of JS), gain experience and new knowledge on real projects.”
In general, there were not many comments of people who want to work in IT, but we still had some. This was our oversight, as there was nothing in the job description about programming. The immediate question was: why did they apply for the position if they plan to develop in a completely different direction? In search of an answer to this question, I suggest comparing the job descriptions of a data annotation project manager and a project manager without additional specifications.
By the results of the analysis of the questionnaires and cases, all applicants were divided into three categories:
- Red (definitely not progressing further) — 155
- Yellow (50/50) — 70
- Green (suitable and demonstrated excellence in cases, given priority) — 70
We didn’t have time to send the CVAT test task to the candidates when we received a huge wave of feedback that CVAT with open access does not work for many due to technical reasons. Some candidates, of course, were able to complete the task, or more creatively, did it in another program with similar functionality! When inviting for an interview, recruiters took this into account, but since it was not possible to create identical conditions for everyone, we decided not to assess the task using CVAT.
To determine the priority of interview invitations, resume screening was conducted. Our colleagues might be surprised: “Screening at the penultimate stage, not at the very beginning!” This is because a data annotation project manager is a new profession, and suitable candidates may have never worked with annotation but have great potential due to their unique background and experience in various fields. When evaluating candidates, the focus was more on a person’s development vector and career goals in the field of data annotation. When it came to prioritizing interviews, attention was also paid to the candidate’s past and additional skills.
As a result of the screening, the first 25 applicants were invited for an interview with HR and operational managers. Three intense days of interviews followed, focusing on candidates’ skills, motivation, growth prospects, and values. Some applicants again shared the story of “wanting to get into IT and not caring about the specific role”—unfortunately for the candidates, these were stop factors. At the end of this stage, 5 offers were made, and all were accepted.
In the end, the hiring funnel for the project manager position looked like this:
- 308 — responses (100%)
- 295 — filled out the questionnaire (95.8%)
- 70 — passed the questionnaire (22.7%)
- 25 — interviews (8.12%)
- 5 — offers (1.6%)
As you can see, a significant conversion slice occurred precisely at the questionnaire stage due to the large number of irrelevant responses.
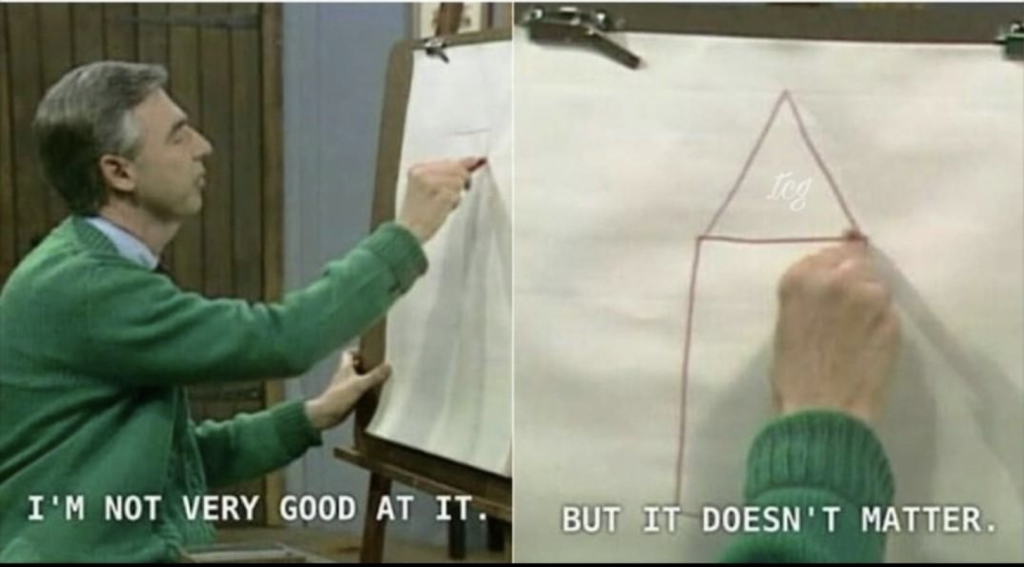
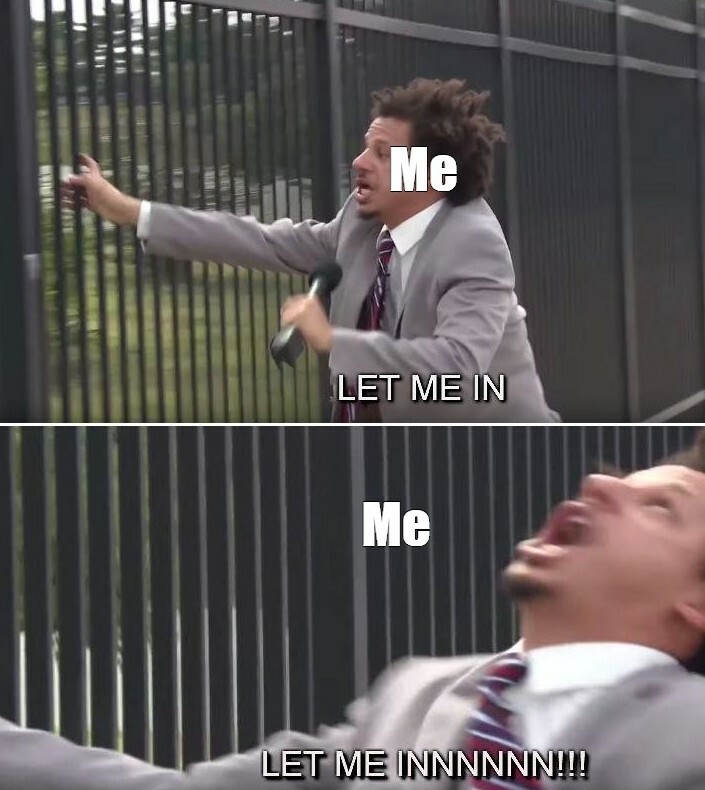
Analyzing results
Perhaps applicants saw an enchanting cat on the TrainingData website and applied. Or, hoping to start a career in IT, they were looking for all possible ways to get there, and data annotation seemed like a short path to the IT world, especially without programming knowledge.
The second assumption seems more realistic. People enter IT from completely different fields and at different ages due to economic necessity. The most obvious fact: IT sector salaries look much more attractive than in other professional areas. Also, people transition to IT during COVID-19 times is driven by the demand for specialists to organize new business processes. For example, in 2020, the number of software developers increased by 12%, reaching 200,000 people.
But in 2022, the picture is not as optimistic — demand for IT specialists has dropped by 25%. The story is fueled by numerous success stories in IT with zero experience and a plethora of advice with the coveted answer to the question: “How to get into IT?” Some authors try to debunk the illusions of IT newcomers, but for the most part, unrealized desires of people are a marketing tool for many schools selling Introduction to Programming with Scratch. People even write entire articles explaining IT memes.
Unfortunately, we mostly receive a large influx of unqualified applications in the labor market in the IT sector.
On the one hand, it is pleasing that people aspire to analyze and make choices in favor of innovative technologies. On the other hand, a question arises: do people have an understanding of where they are aiming and why?
Data collection and annotation for machine learning tasks is a dynamically developing field that requires active and ambitious specialists. Those who are ready to dive into a complex narrow niche and become a real, and most importantly, sought-after expert. TrainingData is open to communication and training for people from completely different fields who aspire to make the world safer and more technological, see their development in data annotation for machine learning, and want to conquer this new, still relatively unknown niche.