TEXT LABELING SERVICES
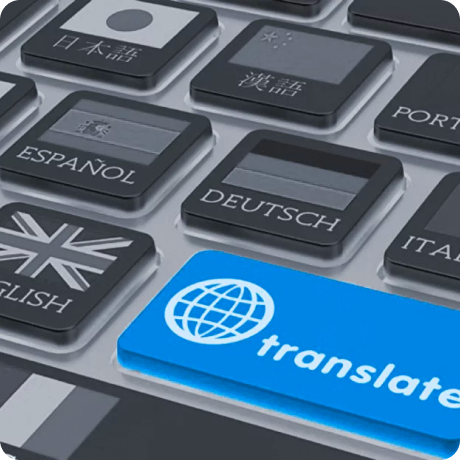
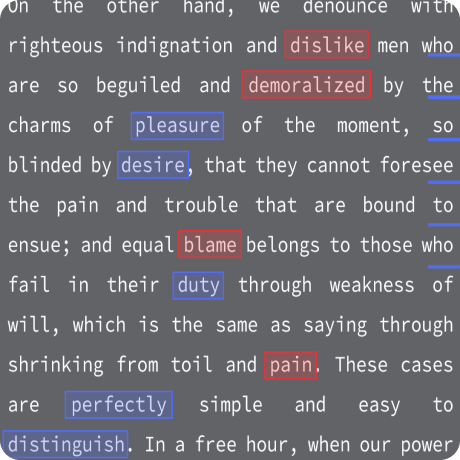
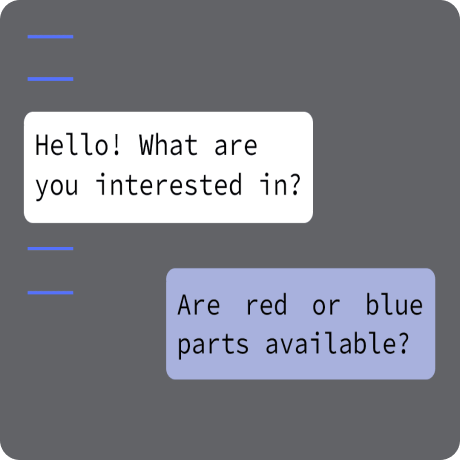
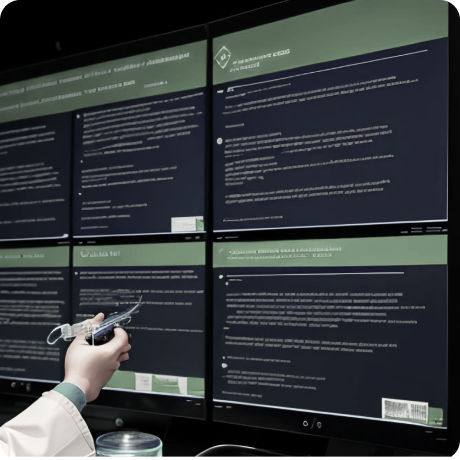
What is Text Labeling?
Types of Text Labeling Services
How we Deliver Text Labeling Projects
At Training Data, we follow a systematic approach to deliver Text Labeling Projects with precision, accuracy, and efficiency. Our process comprises several key stages, each meticulously designed to ensure high-quality annotations and client satisfaction.
Text Labeling Use Cases
Customer Support and Chatbots
Companies use text labeling data to train chatbots and virtual assistants to understand and respond to customer inquiries, complaints, and requests. Labels such as intents, entities, and sentiments enable chatbots to provide personalized and accurate responses, improving customer service efficiency and satisfaction.
Social Media Analysis
Marketing firms leverage text labeling data to analyze social media content for sentiment analysis, trend detection, and brand monitoring. Labels such as sentiment, topics, and user intents enable companies to understand public opinion, track brand perception, and identify emerging trends in real-time.
Market Research and Surveys
Market research companies utilize text labeling data to analyze survey responses, customer feedback, and product reviews for sentiment analysis and trend identification. Labels such as sentiments, themes, and product attributes enable companies to gather actionable insights, identify market opportunities, and make data-driven decisions.
Legal and Compliance
Law firms and regulatory agencies use text labeling data to categorize and analyze legal documents, contracts, and regulatory texts for compliance monitoring and risk assessment. Labels such as legal clauses, contract terms, and regulatory requirements enable companies to identify potential risks, ensure compliance, and mitigate legal liabilities.
Content Moderation and Safety
Online platforms employ text labeling data to moderate user-generated content for inappropriate or harmful material, such as hate speech, spam, and abusive language. Labels such as toxicity levels, offensive language, and content categories enable companies to maintain a safe and inclusive online environment for users.
E-commerce and Product Reviews
E-commerce platforms use text labeling data to analyze product reviews, ratings, and user comments for sentiment analysis and product categorization. Labels such as product features, user sentiments, and review ratings enable companies to understand customer preferences, improve product offerings, and optimize marketing strategies.
Medical and Healthcare
Healthcare providers utilize text labeling data to analyze electronic health records (EHRs), clinical notes, and medical transcripts for information extraction and decision support. Labels such as medical conditions, treatments, and patient demographics enable clinicians to access relevant patient information quickly, improve diagnosis accuracy, and personalize treatment plans.
Human Resources and Recruitment
HR departments use text labeling data to analyze resumes, job descriptions, and candidate profiles for talent acquisition and recruitment purposes. Labels such as skills, qualifications, and job roles enable companies to match candidates with suitable job opportunities, streamline the hiring process, and identify top talent effectively.
Financial Services and Fraud Detection
Financial institutions leverage text labeling data to analyze transaction records, financial statements, and customer communications for fraud detection and risk management. Labels such as suspicious activities, transaction types, and account statuses enable companies to detect fraudulent behavior, prevent financial losses, and ensure regulatory compliance.
Education and Academic Research
Educational institutions use text labeling data to analyze academic papers, research articles, and educational materials for content categorization and knowledge extraction. Labels such as topics, concepts, and citation types enable researchers and educators to organize information, identify research trends, and facilitate knowledge dissemination.
Stages of work
-
Application
/01Leave a request on the website for a free consultation with an expert. Th e acco unt manager will guide you on the services, timelines, and price -
Free pilot
/02We will conduct a test pilot project for you and provide a golden set, based on which we will determine the final technical requirements and approve project metrics -
Agreement
/03We prepare a contract and all necessary documentation upon the request of your accountants and lawyers -
Workflow customization
/04We form a pool of suitable tools and assign an experienced manager who will be in touch with you regarding all project details -
Quality control
/05Data uploads for verification are done iteratively, allowing your team to review and approve collected/annotated data -
Post-payment
/06You pay for the work after receiving the data in agreed quality and quantity
Timeline
-
24 hoursApplication
-
24 hoursConsultation
-
1 to 3 daysPilot
-
1 to 5 daysConducting a pilot
-
1 day to several yearsCarrying out work on the project
-
1 to 5 daysQuality control
in the established quality and quantity
Why
Training Data
- Quality Assurance:
-
Enhanced Data Accuracy
-
Consistency in Labels
-
Reliable Ground Truth
-
Mitigation of Annotation Biases
-
Cost and Time Efficiency
- Data Security and Confidentiality:
-
GDPR Compliance
-
Non-disclosure agreement
-
Data Encryption
-
Multiple data storage options
-
Access Controls and Authentication
- Expert Team:
-
6 years in industry
-
35 top project managers
-
40+ languages
-
100+ countries
-
250k+ assessors
- Flexible and Scalable Solutions:
-
24/7 availability of customer service
-
100% post payment
-
$550 minimum check
-
Variable Workload
-
Customized Solutions
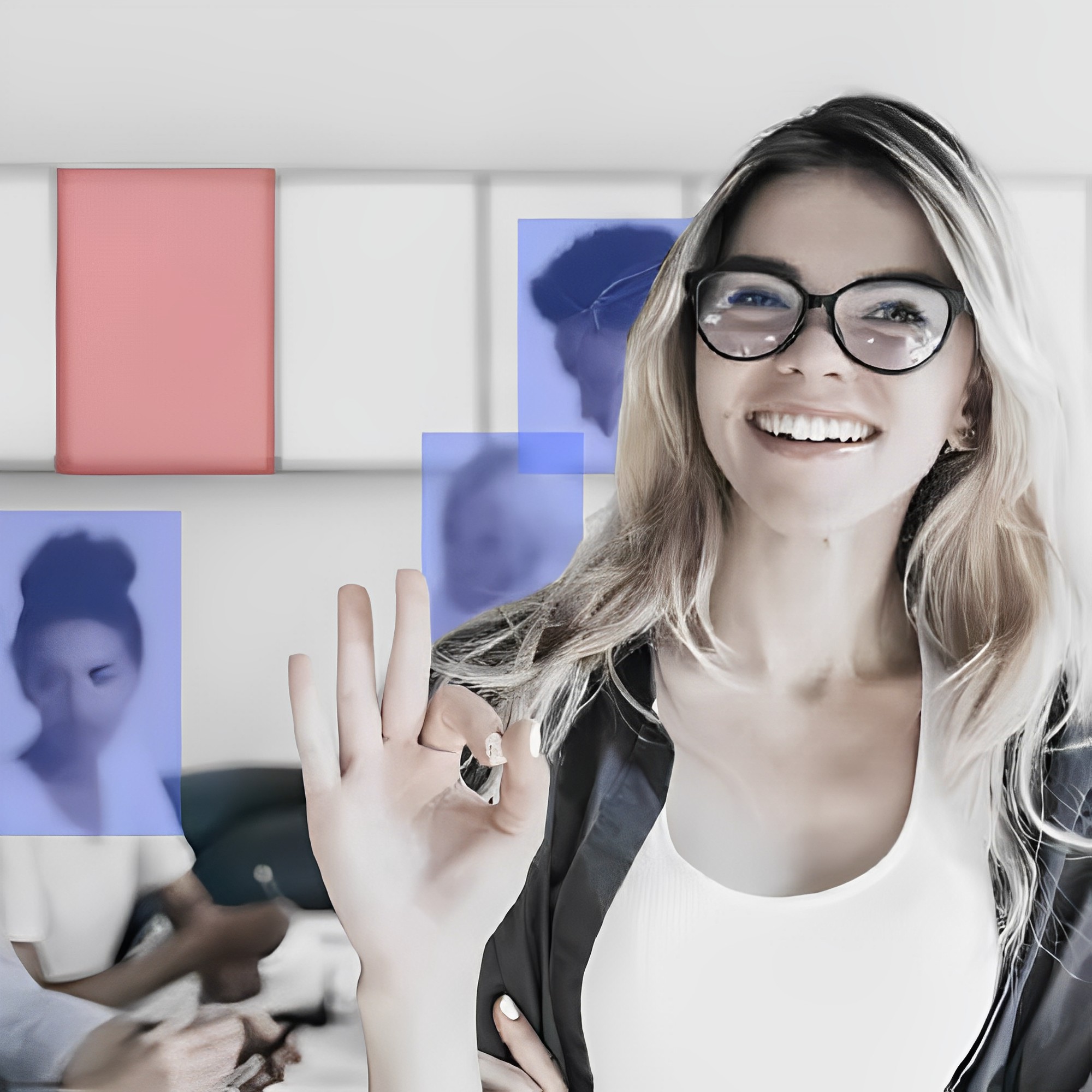