Who is a Data Annotator and What is their Role in ML and AI?
Introduction to data annotation
What is data annotation?
Data annotation is the task of attaching tags to data in order to assist machine learning (ML) algorithms in comprehending it. This process encompasses tasks such as tagging images, categorizing text, and transcribing audio files.
The objective is to furnish machine learning models with annotated data that aids them in recognizing patterns, making decisions, and interpreting information in a manner close to humans.
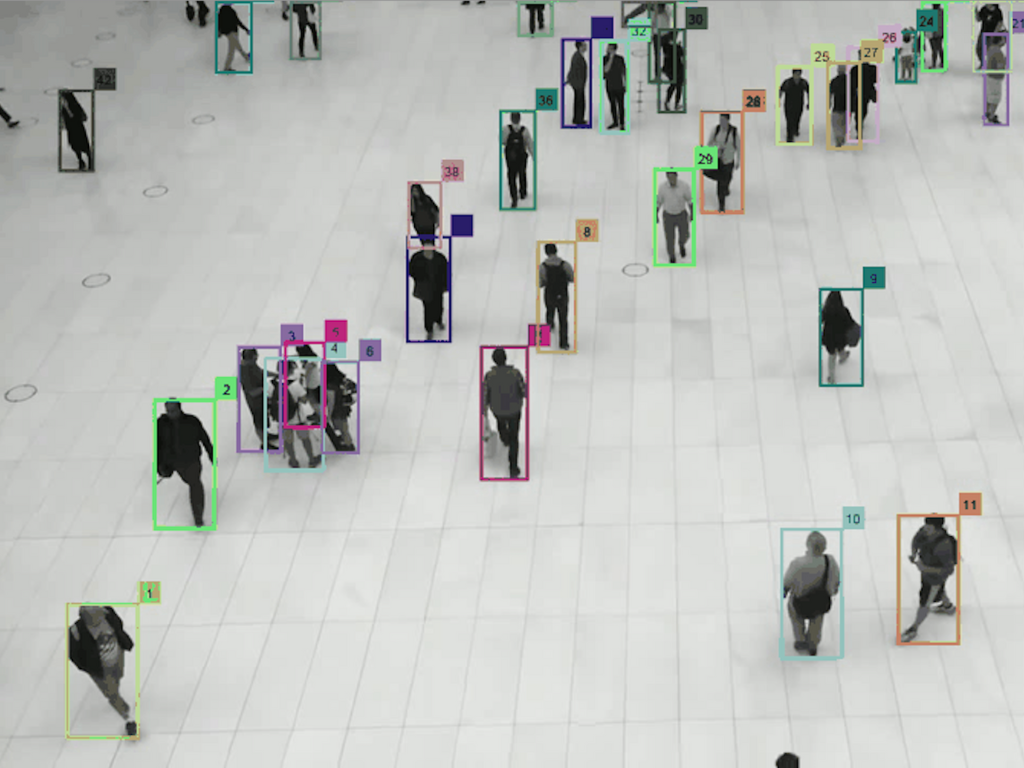
What role does data annotation play in ML and AI?
Data annotation serves as the foundation for training, testing, and refining algorithms that empower ML models and AI systems.
Training machine learning models
The function of data annotation within ML is to provide structured annotated datasets that enable ML models to learn. In the realm of ML annotated data serves as a roadmap for the model by offering instances of input-output relationships.
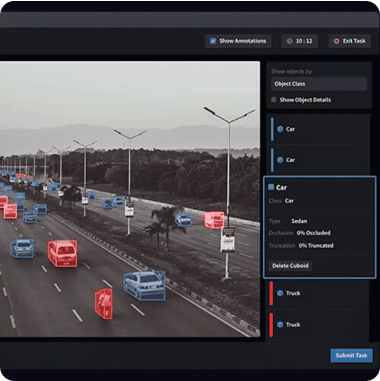
Enhancing Model Precision
The accuracy of machine learning and artificial intelligence models heavily depends on the quality of the data used for training. Detailed and precise annotations reduce uncertainties and errors in model’s predictions. Utilizing annotated datasets during training can boost detection accuracy by 30%.
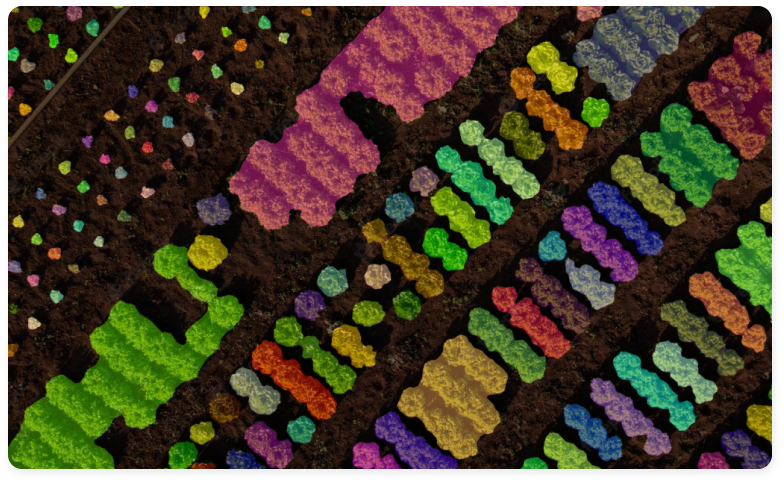
Enabling Context Understanding
Adding specificity and understanding context is essential for AI systems to function effectively in real-world situations. Data annotation plays a role in integrating these aspects into the data.
Annotated data assists AI models in recognizing objects or entities, enabling them to understand the context those objects are part of. This holds importance in natural language processing (NLP) and computer vision where understanding language nuances and visual clues is essential.
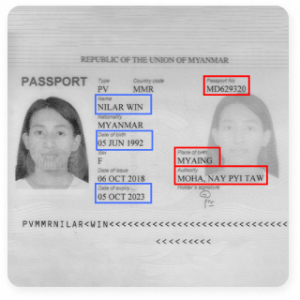
Supporting Continuous Learning and Enhancement
Continual training and optimization aid AI and ML models in adapting to data inputs and evolving real-world circumstances. Data annotation serves as a component of this learning process, providing a feedback mechanism for models to learn from mistakes, adapt to variations, and improve gradually.
Who is a Data Annotator?
A data annotator is an individual tasked with manually annotating data to ensure it’s comprehensible and useful for machine learning models.
Playing a crucial role in the AI and ML processes, these experts ensure that the data provided to machine learning systems is accurate and of top-notch quality. This, in turn, significantly impacts the efficiency and reliability of the AI models.
The Job of a Data Annotator
Data annotators are tasked with examining and tagging data that comes in various forms – such as text, images, videos, or audio. For example, in image annotation tasks this could involve identifying and highlighting elements within an image to help AI systems identify them in varied contexts.
Similarly, when dealing with text data, annotators might categorize content according to its sentiment, or tag text with appropriate syntactic information. Depending on the ML approach, human annotators may also participate in validating model outputs and identifying recurring patterns within datasets.
Overall, data annotators’ responsibilities include:
- Identification and tagging of specific entities in video, text, images and audio data
- Categorization of data/documents
- Model’s output validation
- Identification of common patterns in datasets
Professionals who annotate data are highly sought after across all the digitized industries. Moreover, crowdsourced human annotators are at the forefront of the global AI movement with the invaluable support they offer.
For example, Toloka claims that crowdsourcing can scale up quickly to handle large datasets, with thousands of annotators working simultaneously across the globe.
Educational Background and Skills
While there is no requirement for an educational background to become a data annotator, having a deep understanding of the data content and the relevant field is essential. Employers often prefer candidates with bachelor’s degrees in fields related to data annotation, AI, or machine learning:
- a background in computer/data science or mathematics can serve as a foundation for comprehending the intricacies of the job;
- proficiency in using data annotation tools and software along with a grasp of the data’s subject matter is, of course, essential.
Data annotators need to have a mix of analytical and communication skills. They should be detail-oriented and follow annotation guidelines closely. Also, having critical thinking and domain knowledge are crucial for accurate annotation.
Effective communication and time management, an understanding of quality assurance, independent problem solving abilities – these skills empower annotators to contribute to top-notch datasets for machine learning purposes.
Industries Where Human Annotators Work
Data annotators work in industries where artificial intelligence and machine learning are integral to operational processes. These professionals play a role in the technology and software development field by enhancing algorithms used in search engines and advanced recommendation systems.
- In the healthcare sector human annotators help annotate images and medical records to improve AI driven tools and treatment planning solutions.
- The automotive industry relies on annotators to carefully tag images and data for training driving systems.
- In finance data annotators contribute to detecting fraudulent activities and analyzing large amounts of customer data to forecast financial trends.
- In Natural Language Processing (NLP) annotators categorize text data for sentiment analysis, named entity identification, machine translation, and other NLP goals.
- In the gaming industry annotators focus on video and audio data to improve gaming experience through tasks such as recognizing gestures, speech, and facial expressions.
- Within agriculture annotators tag satellite images and sensor data for tasks like monitoring crops, predicting yields, and detecting pests.
Responsibilities of data annotators
What tasks can annotators perform?
Main duties
Tagging
Data annotators are responsible for tagging tasks that involve not only identifying objects in a dataset, but also understanding and encoding their relationships.
For instance, when observing a picture of a street scene, a human annotator would tag not only cars and people – they would consider contextual relationships such as distance, interactions, and potentially hazardous situations. This deep understanding is crucial for developing AI models that can analyze diverse scenarios and make informed decisions.
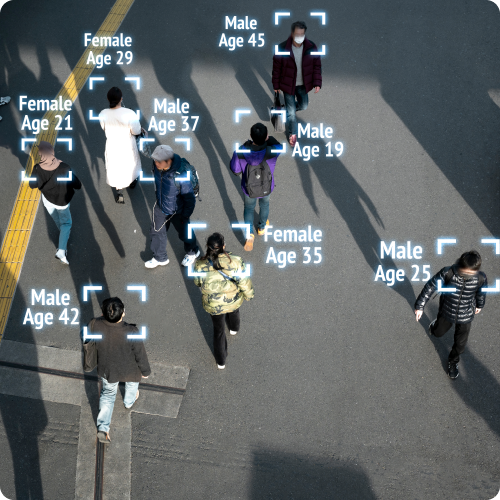
Detailed Classification and Categorization
When it comes to classifying and categorizing data, an annotator’s duties involve organizing information into hierarchies. Instead of just sorting items into general groups they meticulously break down data into finely-tuned categories that capture nuanced differences between them.
This level of accuracy is essential for AI systems that require precise assessments – e.g., distinguishing between types of a specific disease in medical imaging.
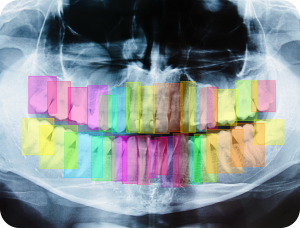
Comprehensive Segmentation and Annotation
Segmentation and annotation tasks performed by data annotators involve creating metadata for elements within datasets. This could involve dividing text into sections or marking images down to the pixel level to help AI in object detection and scene reconstruction.
This meticulous approach to annotation is crucial for training AI models in tasks that demand a high-resolution understanding.
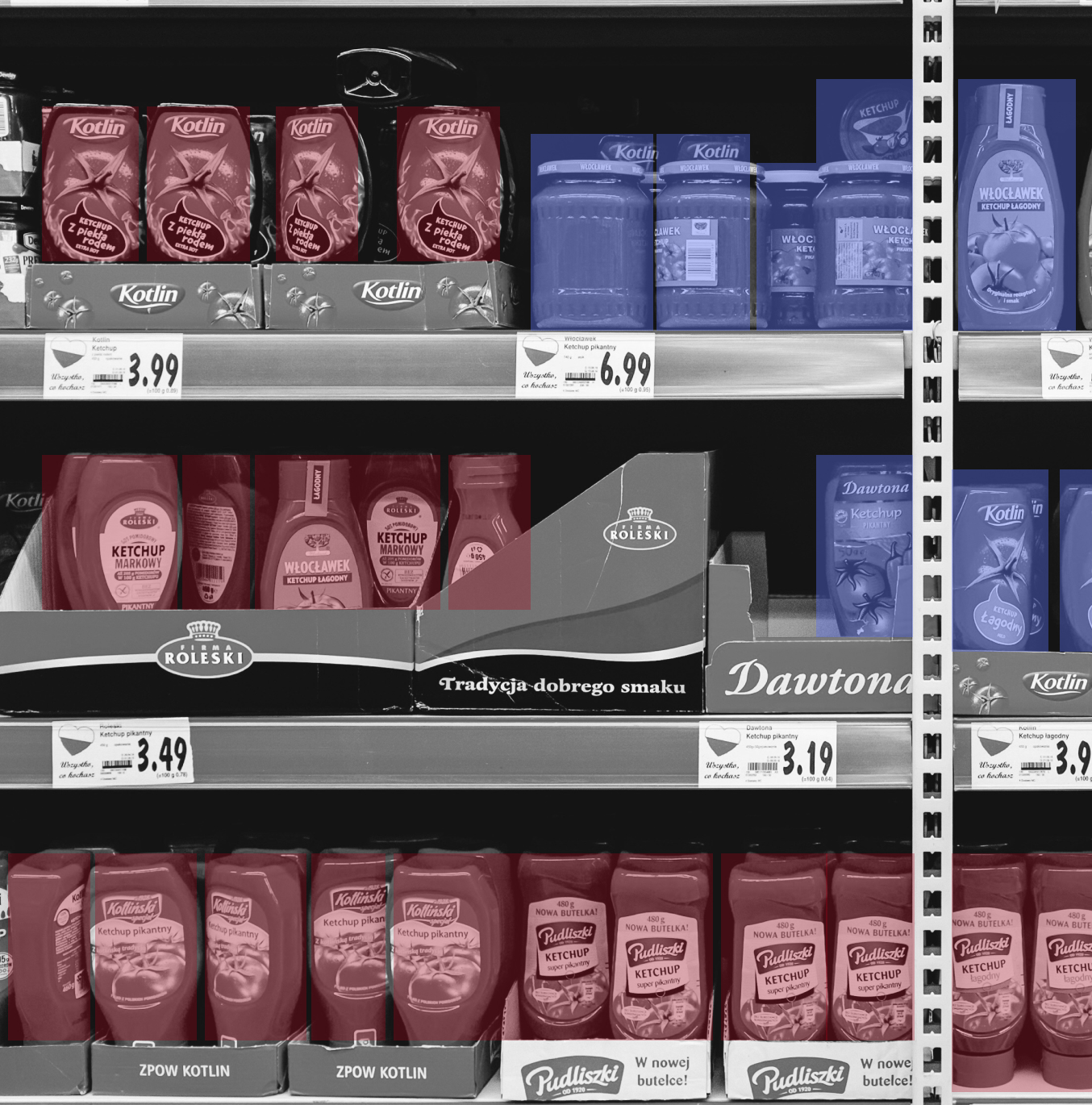
Integration of Multimodal Data
Annotators frequently deal with multimodal data, integrating information from various sources – text, images, audio, and video – to develop extensively annotated datasets. This is critical for the progress of AI systems in handling data streams needed for surveillance or interactive educational platforms.
Dynamic Data Interpretation and Synthesis
Human annotators synthesize data in real time, adjusting to new information and evolving datasets. They consistently update annotations to incorporate new insights and discoveries, making sure that AI models are trained on comprehensive data.
Specialized Duties
Domain-Specific Annotation
Annotators are in demand in domains such as healthcare, finance, or legal sectors where their expertise allows for precise data handling. Within each area, they must understand specialized terminology, concepts, and processes to accurately tag and categorize the domain-specific data.
Quality Control and Validation
A key aspect of data annotators work involves maintaining quality control and validation. They carefully review datasets for accuracy, consistency, and completeness. This rigorous quality assurance process helps prevent errors from propagating within AI training datasets.
Ethical Considerations and Bias Reduction
Data annotators also contribute to addressing ethical concerns and reducing bias in AI datasets. Their role includes identifying and mitigating biases in data, ensuring that annotations do not perpetuate stereotypes or discriminatory behaviors.
This requires vigilance towards the diversity and inclusivity of data to create fair and unbiased AI systems.
Types of data annotators work with
We’ve already mentioned the main data types human annotators work with. Now let’s explore what precisely they do with the said data.
- Textual data: dealing with different types of written content from annotating books and articles for in-depth analysis for real-time sentiment tracking.
- Image data: engaging with visual data formats – categorizing X-ray images for precise diagnoses or examining satellite photos for environmental monitoring purposes.
- Audio data: from adding sound bites to music tracks and conversations to advance technologies like emotion recognition and providing automated transcriptions.
- Video data: describing movements and interactions in videos – essential for AI applications in security, entertainment, and educational sectors.
To learn more about data annotation types and the industries they are used in, read our article here.
Comparison of Manual and Automated Annotation
The process of data annotation can be manual, automated, or hybrid. Still, human annotation is often preferred for its quality despite being more time-consuming and expensive.
Aspect | Manual Annotation | Automated Annotation |
---|---|---|
Accuracy | Exceptionally high, captures intricate details | Variable, often overlooks complex points |
Speed | Slower, prioritizes quality | Rapid, processes volumes swiftly |
Cost | More expensive due to skilled labor | Cost-effective for large-scale processing |
Scalability | More suited to specialized, smaller-scale tasks | Ideal for expansive data sets |
Adaptability | Highly flexible, can navigate complex data types | Best for consistent, well-defined tasks |
Emotional Intelligence | Excellent at interpreting nuanced emotional cues | Has a hard time understanding human emotions |
Contextual Understanding | Deep insights into cultural and situational contexts | Limited capability to grasp context |
Challenges data annotators face
While data annotators are key to preparing highly accurate data to teach ML models, their work is filled with challenges that can impact the quality and efficiency of the data annotation process.
Common Obstacles in Data Annotation and How to Deal with Them
Data Ambiguity
One common issue faced by annotators is dealing with data that lacks clarity. This can lead to varying interpretations and inconsistent annotations. Establishing guidelines and conducting training sessions can help reduce ambiguity and ensure consistency in the annotation process.
Managing Large Datasets
The increasing volume of data being generated, estimated at 2.5 quintillion bytes daily, poses a challenge for annotators. Implementing batch processing techniques and leveraging machine learning tools for annotation can streamline tasks and lessen the workload on human annotators.
Ensuring Quality and Precision
Since accurate annotations are essential in AI model development, many organizations employ multi-tier review processes where experienced annotators double-check and rectify annotations to enhance accuracy levels.
Handling Complex Datasets
Specialized data domains such as medical images or legal documents require extensive expertise. Collaborating with industry experts and integrating their knowledge into the annotation process can significantly improve the quality of annotations.
Time Constraints and Productivity
Meeting project deadlines while maintaining high annotation standards is a tough struggle. Effective time management practices and streamlined workflows are essential for overcoming this issue.
The Critical Role of Human Annotators in Training ML Models
How Does Data Quality Affect AI Performance?
As we’ve already mentioned, the quality of data directly affects how well AI performs. For instance, in image classification, using accurately annotated data can increase model accuracy by 10% to 20% compared to poorly tagged data.
Ensuring data is clean, well-annotated, and free from errors is crucial for training accurate and efficient AI models. A recent study observed that data categorized as “low-quality” impacts models to different extents, highlighting that data quality assessment should be closely tied to the specific task or model.
The same report shows that addressing missing data and incorrect annotations can greatly enhance the classifier performance. While automated machine learning (AutoML) tools are effective at handling issues such as duplicates and inconsistencies, they often face challenges when dealing with missing values data. This highlights the role of both accurate annotating and data cleaning initiatives.
How Are Tech Advancements Shaping the Role of Annotators?
Advances in technology are continuously transforming how we approach data annotation. Automation tools and AI driven systems are increasingly utilized in annotation tasks, allowing human annotators to focus on nuanced aspects that require their immediate judgment.
As AI technology progresses, there is an increasing demand for human annotation that can assist in recognizing emotions, cultural nuances, and subtle contextual differences. This broadens the scope and complexity of annotators roles.
Conclusion
Human annotators are the backbone of machine learning processes. They are trusted to provide high-quality annotations that are used to train models – a crucial task in the ever-evolving technological world we live in.