Exploring Trends and Nuances in Data Annotation for Clients
What key trends do you observe in the field of Artificial Intelligence currently, and how do they impact data annotation?
Now we can observe 5 main trends that are shaping the world of AI:
1. Advancements in deep learning: Deep learning continues to push boundaries and redefine what machines can achieve. Deep learning techniques, such as convolutional neural networks (CNNs) and recurrent neural networks (RNNs), are being used extensively for various tasks like computer vision, natural language processing, and speech recognition. These advancements have greatly improved the accuracy and performance of AI models.
CNNs, resembling the web of connections in the human brain, are the architects behind AI’s ability to decipher images and visual data. They enable computers to see and analyze images in ways that were previously thought to be the exclusive domain of human perception. From recognizing familiar faces to identifying objects in cluttered scenes, CNNs are replicating the human brain’s capability to recognize patterns.
RNNs, on the other hand, give machines the power to understand and generate human-like language by processing sequences of data, such as words in a sentence or notes in a song. This has far-reaching implications, from chatbots that hold coherent conversations to translation tools.
2. Generative AI models: Generative models, such as Generative Adversarial Networks (GANs) and Variational Autoencoders (VAEs), have gained significant attention. These models can generate new data by learning from existing datasets. They have applications in data augmentation, content generation, and other areas. These models have transcended mere algorithmic constructs, opening up a realm of possibilities that promise to reshape our digital landscape.
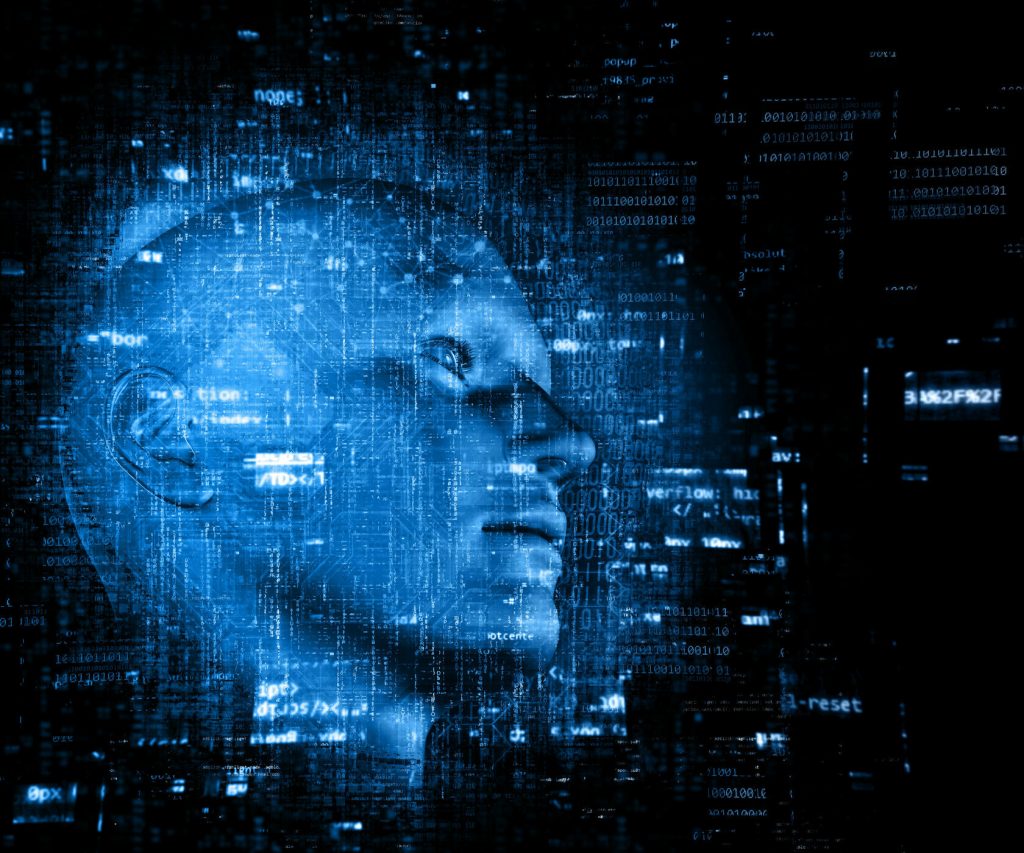
3. Ethical considerations: As AI’s reach extends further into our lives, the discussions surrounding its ethical implications are receiving more attention. Discussions around fairness, accountability, transparency, and privacy are becoming increasingly important. Data annotation plays a crucial role in ensuring fairness and addressing biases in AI models.
4. Edge computing and IoT: There is a growing demand for AI models to run on edge devices with limited resources and connectivity. This trend requires data annotation techniques that can optimize models for efficient inference and adapt to resource constraints.
5. Automated data annotation: As AI applications grow, the need for annotated data also increases. Automated or semi-automated data annotation techniques, like active learning and weak supervision, are gaining popularity to handle large-scale annotation tasks efficiently.
Now we see increasing interest in data preparation for GPT and LLM models. In Training Data we do classification and evaluation of GPT responses for 3 months for different banks, marketplaces, EdTech companies and even search engines.
Which technological innovations or new approaches in data annotation for AI do you find most promising, and why?
In the current landscape, several emerging trends stand out: the exploration of edge cases, which did not exist before, synthetic data markup and pre-markup platforms.
Synthetic data is generated data. Let’s consider a scenario where a client aims to develop a program capable of responding to user inquiries within a chat interface. To do this, you need a comprehensive dataset encompassing diverse chat interactions, – a resource the company lacks. By crafting conceivable dialogues we create synthetic data.
In tandem with synthetic data, the emergence of pre-markup platforms carries significant potential. These platforms exhibit the capacity to expedite and streamline the annotation process. In parallel, synthetic data possesses the ability to enrich the existing dataset’s diversity and relevance, all while contributing to cost reduction.
The landscape of technological advancements also encompasses domains such as Generative AI, Medical AI, edge cases, and biometric data. The state and businesses are facing more and more tasks related to security and healthcare. Thus, ML teams have an increased need for high-quality, diverse data containing personal, sensitive information.
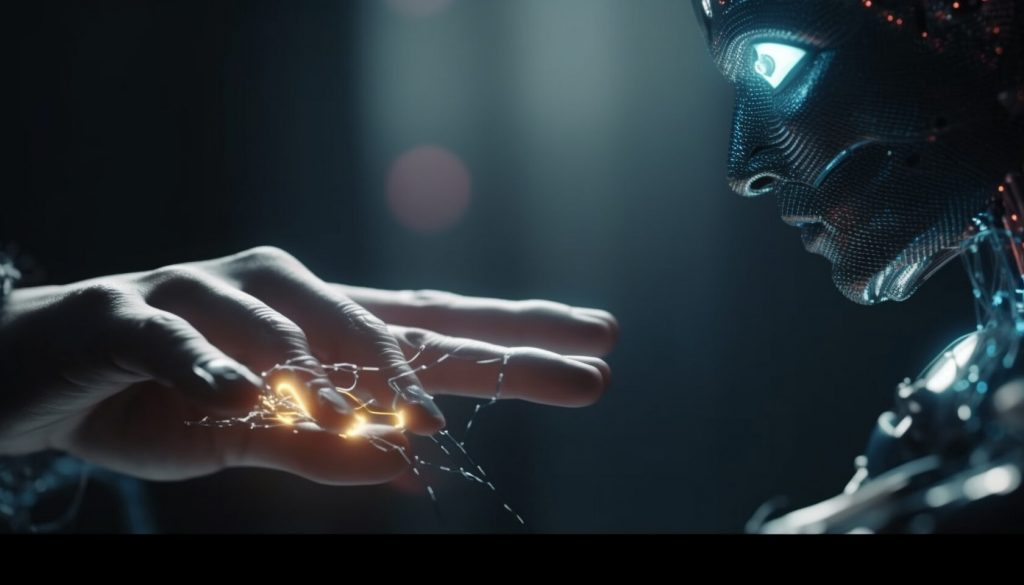
What strategies or techniques do you employ to optimize the efficiency and speed of the data annotation process?
For these purposes, we employ three primary strategies:
1. The Segment Anything Model (SAM) in SVAT is an innovative approach that revolutionizes video analysis. SAM excels at precisely identifying and delineating various objects and segments within videos. With its capabilities, SAM empowers SVAT users to efficiently annotate and comprehend video content.
2. Chat GPT and neural networks of the Mind journey type. We introduce into the work not only assessors, but also managers in different verticals. Neural networks cannot completely replace our work, since the pitfalls, corner cases, the human factor and changing conditions within the project are still being solved by human specialists.
3. Our own neural networks that we develop for internal solutions and for customer projects to optimize metrics.
Can you share any examples of how data annotation has directly improved the performance or accuracy of AI models in your previous projects?
For instance, our customer approached us with a project to improve his system of tracking people by video. The client presented us with an initial dataset, including 100 hours of video footage. Together we achieved a notable advancement of 14% in the metrics.
In another project, we collaborated with one of the top 3 Banks in Eastern Europe. The focus of this engagement was the processing of calls received by their call center. The bank had a vision to harness the potential of neural networks in automating the classification of customer requests into distinct categories such as debit cards, credit, deposits, and balance inquiries.
Leveraging our comprehensive dataset, the bank was able to successfully train a neural network to accomplish this task with an accuracy rate of 96%. As a result, the system exhibited a capability to accurately identify the subject matter of bank-related inquiries. Subsequent interactions with the bank post-project showed a 27% acceleration in the pace of call center operations.
How do you manage client expectations regarding the turnaround time for data annotation projects, considering the increasing demand for AI training data and the need for accuracy?
To manage client expectations regarding the turnaround time for data annotation projects, considering the increasing demand for AI training data and the need for accuracy, the sales team can adopt the following approaches:
1. Clear communication: Establishing effective communication with the client is essential. Explain the challenges and complexities involved in data annotation, such as the amount of data to be processed, the need to ensure data quality and accuracy, and the time required to complete the project.
2. Accurate timeline assessment: It’s important to conduct an accurate assessment of the time required for data annotation. Base this estimation on the current team capacity, data volume, and project complexity. Avoid making unrealistic promises but provide realistic estimates to avoid disappointments.
3. Explore scalability options: To cope with the increasing demand, consider scalability options like expanding the data annotation team or utilizing reliable outsourcing services. This can help meet the client’s demand within the desired timeframe.
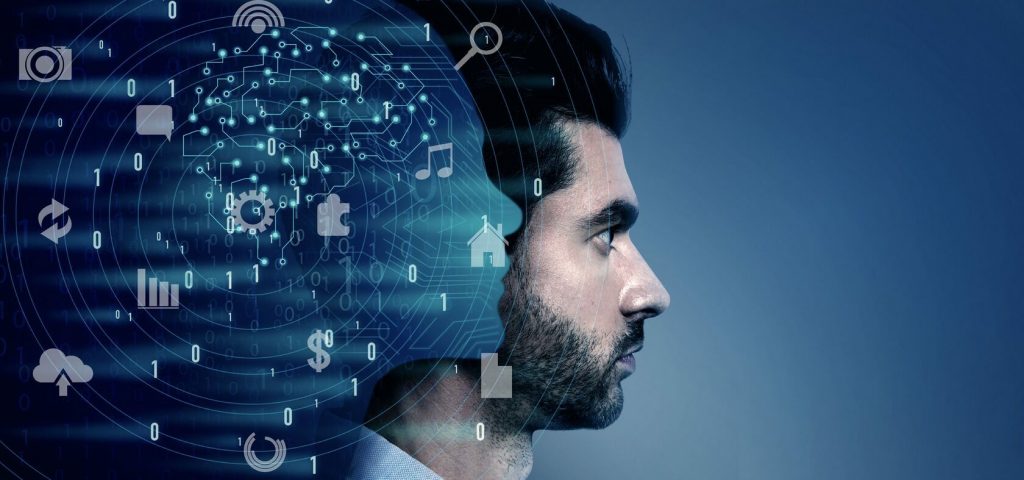
4. Prioritization and workflow management: Establish appropriate priorities for projects by efficiently managing the workflow. This can be achieved through a project management system that tracks progress and helps optimize resource allocation.
5. Continuous transparency: Keeping the client informed about project progress is crucial to managing their expectations. Share regular updates regarding the project status, including any delays or unexpected setbacks.
6. Constant feedback: Establishing a feedback channel with the client can help identify their concerns regarding the delivery timeline and enable process improvements in data annotation to meet expectations.
7. Adoption of automation: Utilize specific automation tools to accelerate the data annotation process, which can improve efficiency and reduce turnaround time. However, quality assurance must be maintained even with the use of automation.
8. Flexibility and adaptation: As client demands and requirements evolve, it’s important to adapt and adjust strategies as necessary. This may involve investing in new technologies, team training, or continuous process enhancements.
By following these guidelines, the sales team can manage client expectations regarding the turnaround time for data annotation projects, ensuring a balance between increasing demand and the need for accuracy.
Could you share some successful strategies or approaches you’ve used to overcome clients’ concerns or objections related to data quality and reliability in Al datasets?
Our clientele consists of innovation and AI technology teams. I find myself needing to emphasize the significance of technology and high-quality data 98% of the time. It is a well-known fact that 80% of the success of neural network training lies in the appropriate quality and volume of data.
Here we developed a strong expertise, because data and all types of work with them have been our specialization for more than 5 years. Training Data, being an enhancement of the client’s ML team, offers the most optimal option for achieving certain project metrics. Collaboratively with our clients, we curate a toolbox, adjust timelines and tasks within the roadmap, and progress in alignment with the agreed-upon plan.
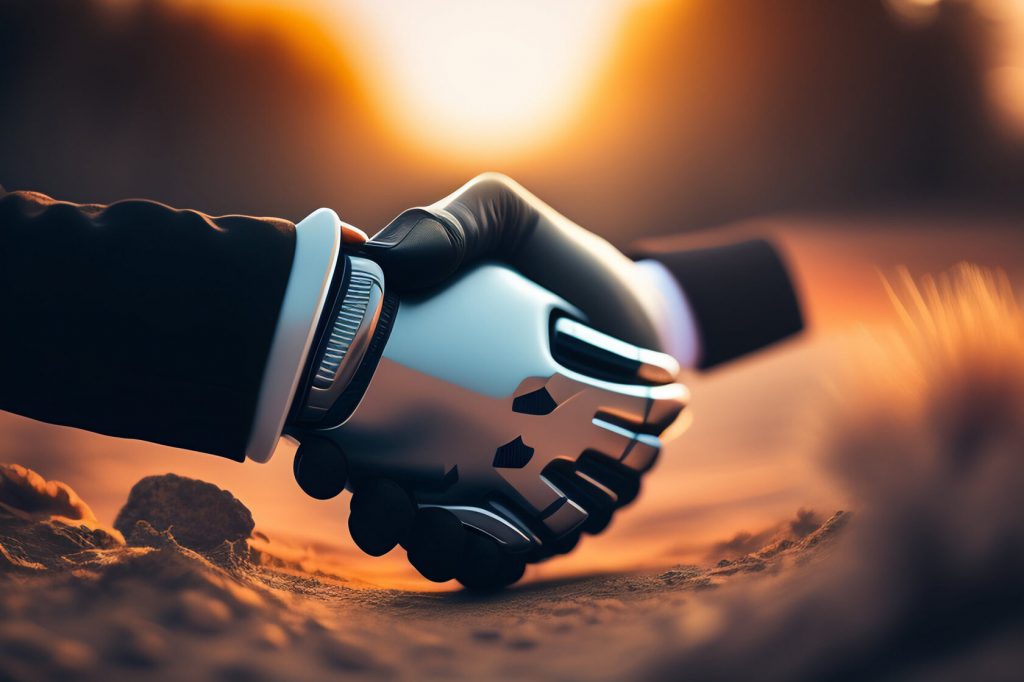
What measures do you take to ensure data security and confidentiality for clients’ sensitive information during the data annotation process?
Our approach encompasses a multi-faceted strategy designed to provide comprehensive protection.
One of the key measures we undertake is the implementation of Non-Disclosure Agreements (NDA). We also bolster security through an array of closing documents that solidify the commitment to data integrity and privacy.
Recognizing the unique requirements of our clients, we offer the flexibility to operate within their own environment. This approach ensures that the data remains within the controlled confines of the client’s infrastructure, enhancing security by minimizing external exposure.
As an additional security measure, all data transmissions occur exclusively through secure repositories.